A new study from the University of Melbourne has demonstrated how hiring algorithms can amplify human gender biases against women.
Researchers from the University of Melbourne gave 40 recruiters real-life resumés for jobs at UniBank, which funded the study. The resumés were for roles as a data analyst, finance officer, and recruitment officer, which Australian Bureau of Statistics data shows are respectively male-dominated, gender-balanced, and female-dominated positions.
Half of the recruitment panel was given resumés with the candidate’s stated gender. The other half was given the exact same resumés, but with traditionally female names and male ones interchanged. For instance, they might switch “Mark” to “Sarah” and “Rachel” to “John.”
The panelists were then instructed to rank each candidate and collectively pick the top and bottom three resumés for each role. The researchers then reviewed their decisions.
[Read: How to build a search engine for criminal data]
They found that the recruiters consistently preferred resumés from the apparently male candidates — even though they had the same qualifications and experience as the women. Both male and female panelists were more likely to give men’s resumés a higher rank.
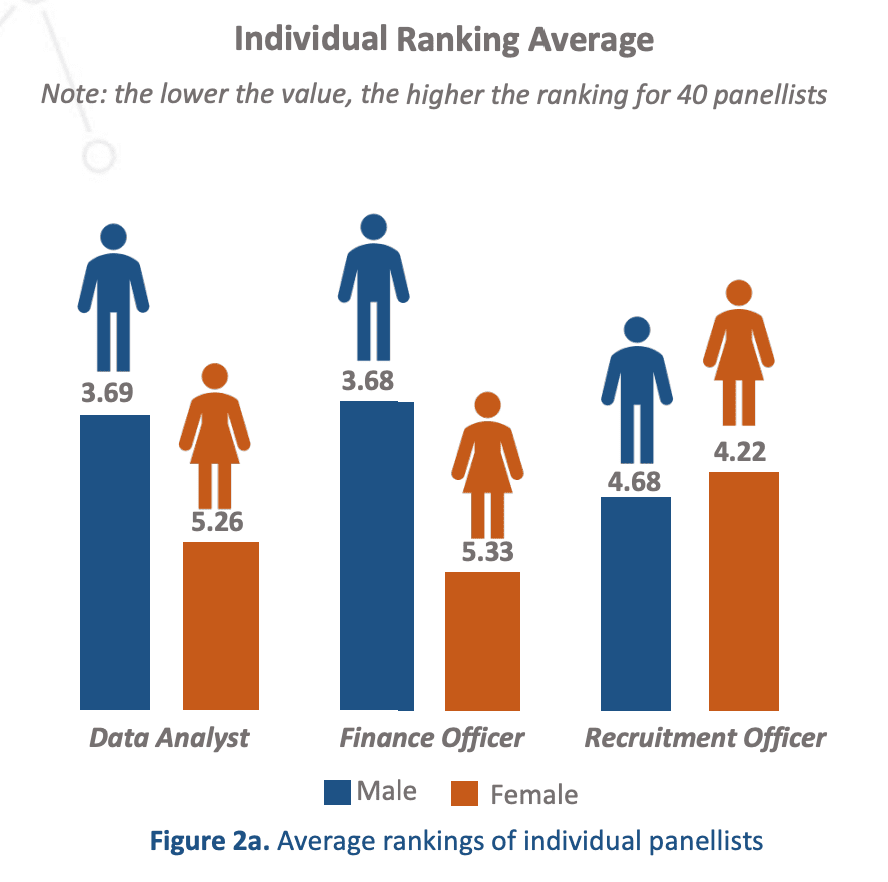
The researchers then used the data to create a hiring algorithm that would rank each candidate in-line with the panel’s preferences — and found that it reflected their biases.
Read: Amazon’s sexist hiring algorithm could still be better than a human
“Even when the names of the candidates were removed, AI assessed resumés based on historic hiring patterns where preferences leaned towards male candidates,” said study co-author Dr Marc Cheong in a statement.
“For example, giving advantage to candidates with years of continuous service would automatically disadvantage women who’ve taken time off work for caring responsibilities.”
The study relied on a small sample of data, but these types of gender biases have also been documented in large companies. Amazon, for example, had to shut down a hiring algorithm tool after discovering it was discriminating against female applicants, because the models were predominantly trained on resumes submitted by men.
“Also, in the case of more advanced AIs that operate within a ‘black box’ without transparency or human oversight, there is a danger that any amount of initial bias will be amplified,” added Dr Cheong.
The researchers believe the risks can be reduced by making hiring algorithms more transparent. But we also need to address our inherent human biases — before they’re baked into the machines.
Published December 2, 2020 — 18:06 UTC