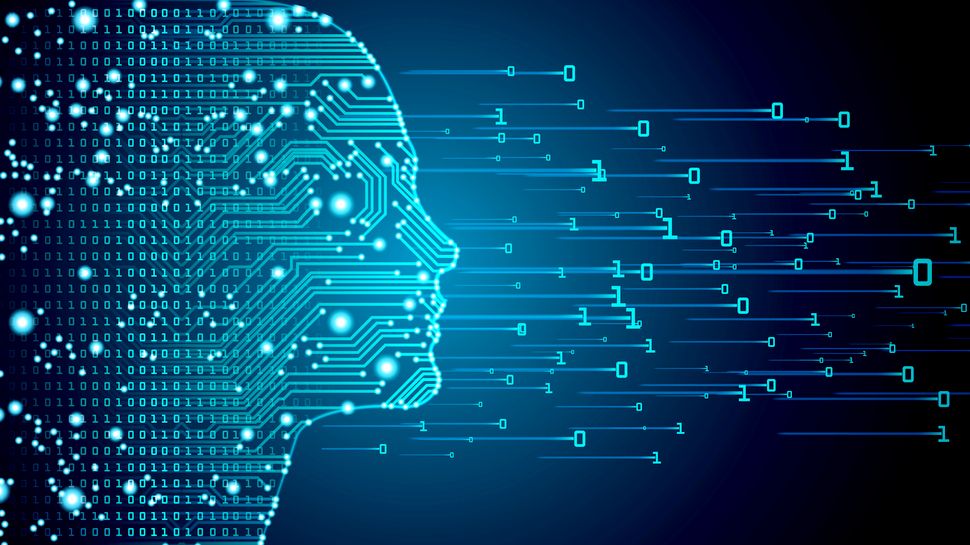
Data can be sourced from anywhere, such as business tools and applications, website analytics, industrial equipment, wearables, social media – its sources are limitless. However, to build a trusted AI system, data must be compliant, accurate, clean, and most importantly, relevant for the intended purpose. Data has to have meaning. It’s the most important thing to build trusted data and build AI at scale. Unreliable data can derive from poor data-collection practices such as customer relationship management (CRM) and enterprise resource planning (ERP) or from call centers, to name a few. Businesses that use practices such as these aren’t necessarily aiming for qualitative data.
To overcome this challenge, it’s imperative that companies build a “business data fabric” and execute a strong data governance, as an integral part of the fabric, to help them monitor the data they use to train AI models. However, our research shows that only 8% of corporate companies have a governance function already established, so this is an area on which businesses need to be advised.
Consequently, AI itself can also be part of the solution to fix data quality, with algorithms being able to extract information from handwritten forms, amend data entry errors and match information across systems. AI can be used to self-discover data, enabling an automated matching between technical terms and business terms, so that data can be meaningful and trusted. Companies will still need human validation checks, but machine learning will “learn” from these human interventions with the system becoming smarter and smarter over time. In this way, businesses can ensure AI applications leverage only trusted data.
In fact, our Trusted AI Platform provides insights to organizations on the sources and drivers of risk and guides an AI design team in quantifying AI risks. By developing a quantitative score of an AI system’s residual risk, the EY Trusted AI Platform calculates the residual risk of an AI system. Based on the anticipated impact on users, stakeholder risk acts as a multiplier to technical risk, considering social and ethical implications. The evaluation of governance and control maturity acts as a mitigating factor to reduce residual risk of an AI system.