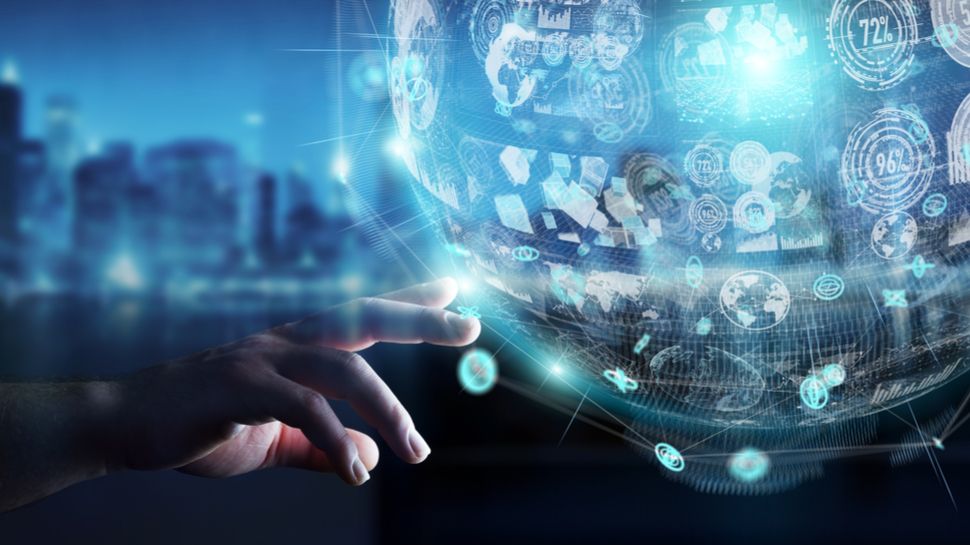
For all the talk about how AI and machine learning will transform business, we have barely scratched the surface of how data science can help businesses day-to-day. That’s because the people who will come up with the most effective applications for data science – the domain experts – aren’t yet involved.
About the author
Andrew Beers is Chief Technology Officer at Tableau.
Every day countless business decisions are made, which would greatly benefit from being data-driven. However, we can’t expect everyone to become a data scientist. We need to make data analytics more accessible and actionable for people, it only needs to be help employees to make faster and more informed decisions. Greater access to data will let business experts get to the last mile of analysis faster and with more confidence, using their judgement to get the rest of the way.
Data science is centralized and out-of-balance
To be transformative, organizations need agility and that comes from making the right decisions fast. But the infinite amounts of data coming in right now are too much for many organizations to handle. At best organizations have a small number of highly technical data experts supporting large teams across the business. Oftentimes these data experts are maxed out working on issues with the highest priority, yet people all across the business still need help to solve contextual problems that require some data science but don’t justify – or can’t wait for – a data scientist. That means critical business decisions are being made daily that aren’t supported by the centralized data science team.
What would more data science in business look like?
For example, taking a data-led approach to marketing and sales with opportunity scoring, predicting time to close, and many other CRM-related use cases are highly valuable to the business. Manufacturers and retailers can benefit from AI-based models for supply chain distribution and optimization, forecasting consumer demand, or exploring scenarios for adding new products to their mix.
Human resources might use data to assess the likelihood of a candidate accepting an offer by analyzing historical patterns and factoring in the recruiter’s knowledge of how they can adjust the mix of salary, equity, benefits, etc., based on the candidate’s values. Corporate real estate could plan where to buy office space using modelling.
Building confidence without coding
To make it possible for every business person to use data in their daily lives, it needs to be accessible without having to learn Python, machine learning, or how to tune parameters for an algorithm. First, we need to value the role that human experience plays in data-driven decision making. Typically, knowing the right question, and knowing what you are going to do with the answer, is more important than details like model selection.
Organizations often spend a lot of effort trying to recruit “unicorns” that have both data science and domain expertise, but people who fit this description are incredibly rare. That’s why we need technology to do the heavy lifting. We need tools that eliminate barriers for people with business context to quickly build models and apply predictions. Data literacy just needs to be good enough to help people get data and get what it can do for them – and data visualization is a great place to start.
Data visualization enables better data literacy
It’s easy for people to understand data immediately when they see it visually, especially when compared to studying a table of numbers. When data is in visual form it is far easier to see outliers, to see trends, and to see details in rich ways – to be able to get answers faster. A well-crafted thoughtful visualization of data can turn on a lightbulb in someone’s head. This has huge benefits when a team will include people with a wide range of data literacy skills. It’s a leveler, which speeds up understanding of data for everyone, feeding into quicker decision-making.
Democratizing access to data analytics leads to faster and more informed business decisions, and will future-proof businesses. With guided AI experiences in the hands of domain experts, teams can apply advanced analytics to more business problems and make important decisions faster and with more rigor, while still leaning into their human judgment. It’s not about fine-tuning super precise models but guiding the people who are closest to the problem in the right direction. We must teach everyone basic data literacy and accept that data science for business experts does not need to be incredibly exact.