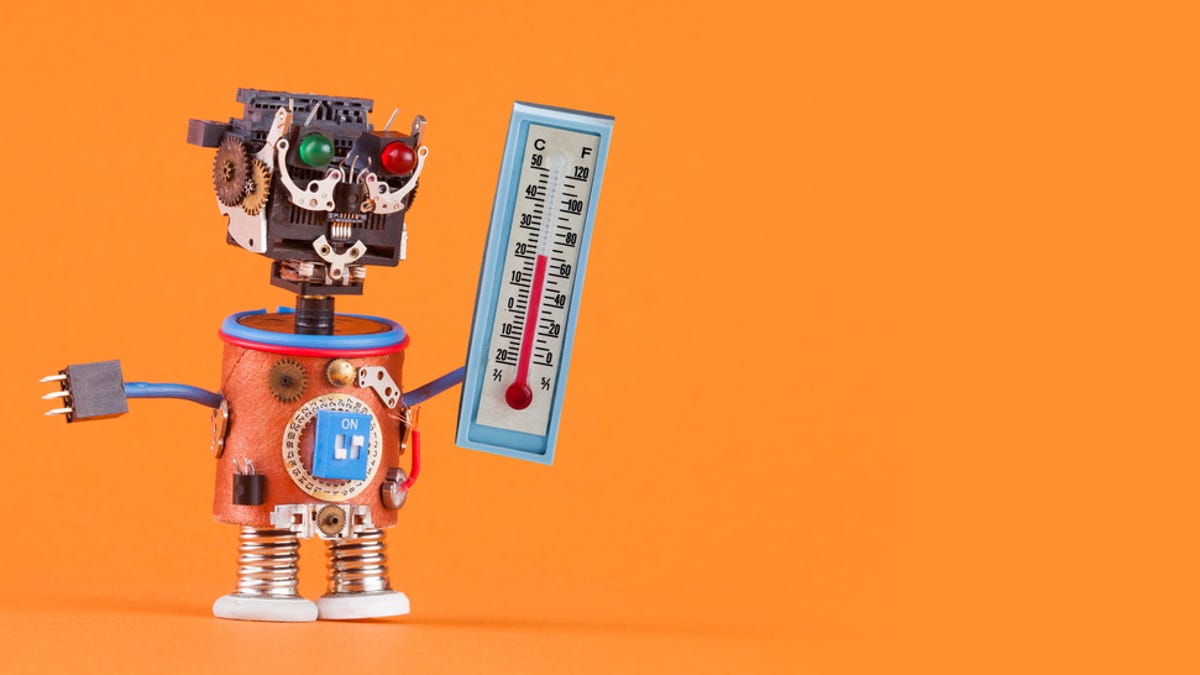
Finally, a robot to tell you which jacket you should wear to the function. Google DeepMind, the search giant’s AI-centric brain trust, just announced a new weather forecasting model that beats traditional systems more than 90% of the time. Named GraphCast, the machine learning model promises 10-day predictions that are better, faster, and more energy-efficient than the tools that run your weather app today.
“We believe this marks a turning point in weather forecasting,” Google’s researchers wrote in a study published Tuesday.
Advertisement
In general, the current model for forecasts is called “numerical weather prediction (NWP).” NWP plugs current weather conditions into enormous models that simulate upcoming changes based on the principles of fluid dynamics, thermodynamics, and other atmospheric sciences. It’s complicated, expensive, and calls for tons of computing power.
Advertisement
Instead of running simulations about how molecules will fly around and slam into each other, GraphCast breaks with tradition by placing a heavier emphasis on historical data. In other words, it’s a machine-learning model that makes predictions based on what happened in the past. There’s a lot of fancy computer science involved, but in general, it’s a lot simpler in terms of the level and number of computations it requires.
Advertisement
GraphCast starts with the current state of Earth’s weather, and data about the weather six hours ago. Then, it makes a prediction about what the weather will look like six hours from now. GraphCast then feeds those predictions back into the model, performs the same calculation, and spits out longer-term forecasts.
The Google team compared GraphCasts results to the current model that’s used for medium-range weather prediction, called HRES. According to the study, GraphCast “significantly” outperformed HRES on 90% of the targets used in the test.
Advertisement
GraphCast also had surprising success predicting extreme weather events including tropical cyclones and freak temperature changes, even though it wasn’t specifically trained to handle them.
The study authors say their work is meant to work alongside the standard systems meteorologists rely on. “Our approach should not be regarded as a replacement for traditional weather forecasting methods,” the study authors wrote. “Rather, our work should be interpreted as evidence that [machine learning weather prediction] is able to meet the challenges of real-world forecasting problems and has potential to complement and improve the current best methods.”
Services Marketplace – Listings, Bookings & Reviews