This article was originally published by Federico Cheli on Cities Today, the leading news platform on urban mobility and innovation, reaching an international audience of city leaders. For the latest updates follow Cities Today on Twitter, Facebook, LinkedIn, Instagram, and YouTube, or sign up for Cities Today News.
Artificial intelligence (AI) applications in the transport sector are stimulating innovations for better and more targeted use of vehicles and infrastructure. This could optimize network performances, support the monitoring and management of traffic, and create the base for solutions that pave the way to future mobility, particularly in cities.
Infrastructure management and vehicle design are evolving thanks to the opportunities offered by the widespread use of devices, such as smartphones and in-vehicle localization sensors, for processing, gathering and exchanging information among users and service providers, as well as for monitoring and detecting the performance of vehicles and behavior of people.
Altogether these create a huge amount of data (big data), which is the primary source for using AI in transport, allowing computers to perform activities for humans, such as driving.
Levels of automation
The most advanced and revolutionary AI application in transport today is the automation of vehicles. The classification most used to describe the degree of automation is defined by standards from the International Society of Automotive Engineers (SAE). This provides six levels of automation (including Level 0, which means there is no automation), identified according to who (i.e. human driver or system) performs the operation and at what time.
Today’s cars are generally equipped with SAE level 1 and 2 features, commonly referred to as Advanced Driver Assistance Systems (ADAS), such as park assist, cruise control, adaptive front lights and lane keeping assist (see figure 1). These devices support drivers in terms of providing aid, warning and assistance, rather than replacing them in driving activities (full automation).
In addition, a few vehicle manufacturers can offer models furnished with partial automation functions, such as autopilot under certain conditions and autonomous valet parking, but drivers are required to be in control of the vehicle at all times (SAE level 3).
In future, leading car manufacturers, as well as newcomers in the automotive industry such as Google, are expected to commercialize fully automated vehicles (AVs), which will be able to act as an intelligent agent and to adapt to their context using data collected in real-time from cameras, Light Detection and Ranging (LiDAR) systems, localization sensors and digital maps.
Adapting infrastructure
Although some may argue that AVs will be able to operate in any external context, it is very likely that AI will also impact the way that transport infrastructure is designed and managed, in order to improve safety conditions and to control traffic conditions in real-time.
Future mobility will be characterized by an extensive use of vehicles requiring a continuous dialogue with other elements of the system (vehicles, pedestrians, cyclists and the infrastructure) to orient themselves in the driving environment, i.e. connected and automated vehicles (CAVs) and by ‘smart roads’ adequately equipped to accommodate them. Intelligent traffic lights are an example of how vehicles and infrastructure could cooperate both to improve overall traffic conditions by adapting the green/red cycles to the actual flows approaching the intersection, and to prioritize vehicles, such as ambulances, recognizing an emergency by processing data, sounds and images.
Such technological advances in vehicles and infrastructure will also be accompanied by the development of innovative transit services to promote the use of seamless multimodal transport solutions. If correctly planned and integrated with existing mass rapid transit, CAVs could lead to great benefits in terms of environmental, social and economic sustainability, promoting a more efficient and conscientious use of resources.
For example, self-driving vehicles could primarily be used as “feeder services” to connect people living in rural areas or in low-density urban zones – where transit lines do not operate with high frequencies due to low demand levels –to metro and train stations, enlarging their catchment areas, increasing public transport patronage and reducing the need to own a car.
They could also enhance the potential mobility of users with limited capabilities or without a driving license (elderly or young people, for instance), and allow them to carry out other activities, such as reading or talking on the phone, while traveling by car.
It is predicted that automated systems will also feature heavily in freight transport, both for first and last-mile delivery in urban areas, through autonomous light commercial vehicles, or ‘road drones’.
The road ahead
In fact, it is likely that some radical changes in urban transport will occur in the near future. Travellers will be provided with mobility solutions for their trips which do not require vehicle ownership but are based on renting and sharing with the rest of the community (i.e. shared mobility). However, the wide diffusion of vehicles in urban areas could lead to an increase in road congestion, higher energy consumption, polluting emissions, visual impact and land use expenditure. A higher volume of trips might be generated not only by the availability of more vehicles but also by the presence of empty vehicles running on roads to avoid parking or to send driver-free deliveries.
In conclusion, it is necessary to carefully assess the broad spectrum of potential impacts of AI applications on transport systems against the changes they will bring to mobility, considering also the impact on land use, social inclusion and more.
Cities should prepare for these changes with a two-fold approach. In the short term, they could invest in the technological innovations that become available on the market and integrate them into the existing systems. This is already happening with intelligent traffic lights, for example. These devices are capable of self-regulating the red-green cycle according to the flows approaching the intersections and they can self-program according to time of day, week and month.
At the same time, cities will have to plan long-term future mobility with a visionary approach that captures the extent of the innovation that awaits us and that will be characterized by infrastructure and vehicles communicating with each other (Vehicle-to-Infrastructure/V2I) and with people (Vehicle-to-People/V2P), monitoring themselves and automatically reacting to external conditions.
Cities must plan a smooth transition towards the future – an economically sustainable transition but also an inclusive one, avoiding any form of digital divide. This means rethinking mobility systems, including public transport services, infrastructure maintenance, logistics, fares and regulation. It also means investing in capacity-building and training in order to educate and set the standard for high-level transport analysts to be able to manage and govern these new processes.
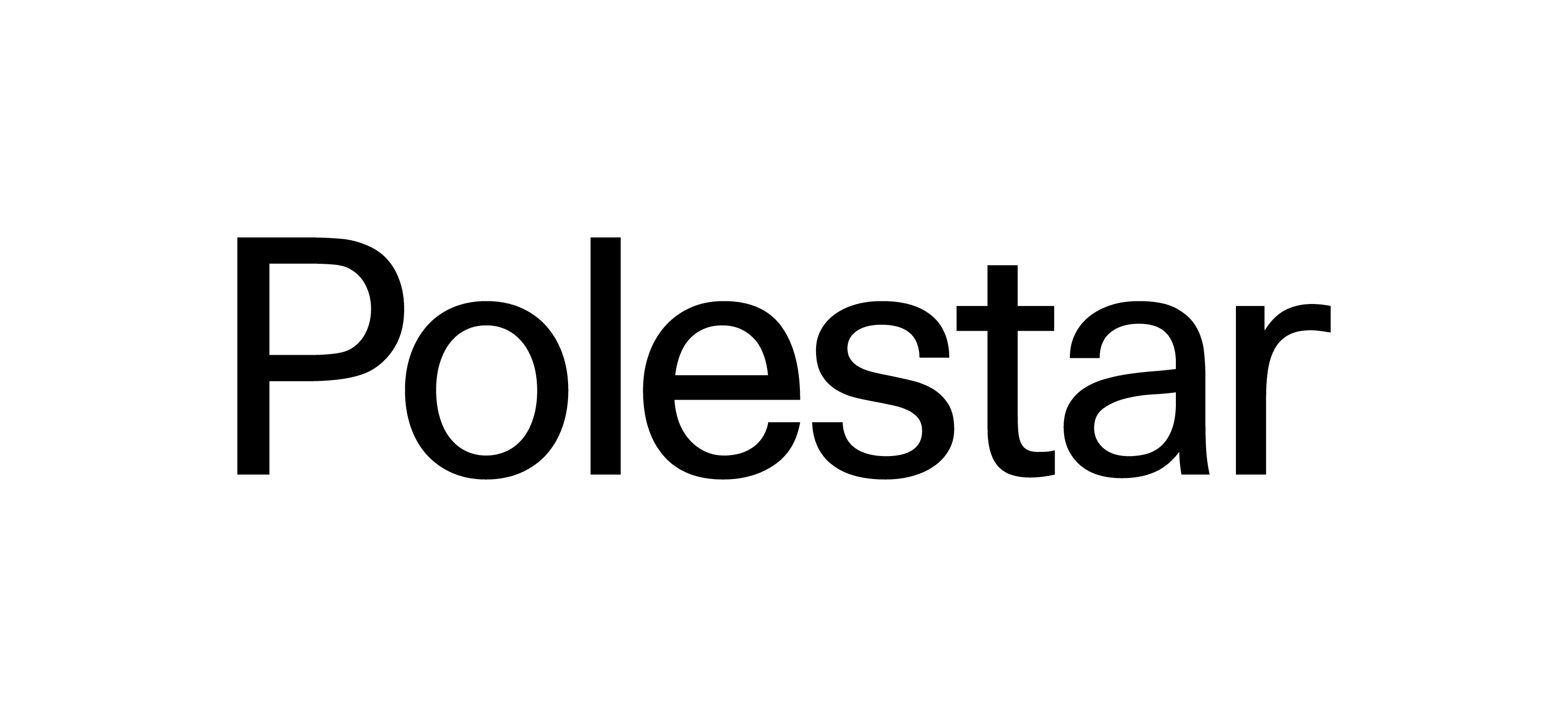
SHIFT is brought to you by Polestar. It’s time to accelerate the shift to sustainable mobility. That is why Polestar combines electric driving with cutting-edge design and thrilling performance. Find out how.
Published November 4, 2020 — 10:00 UTC