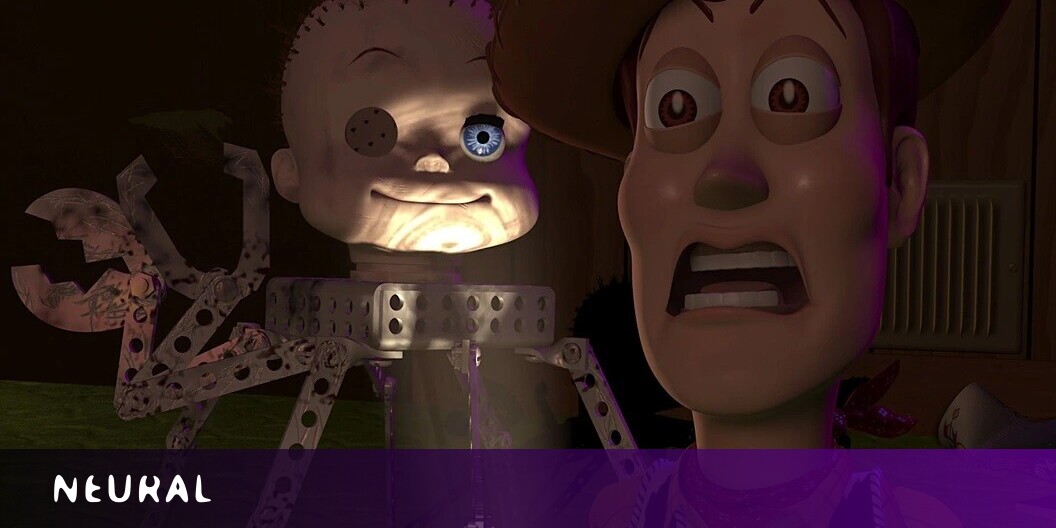
Artificial intelligence may be the most powerful tool humans have. When applied properly to a problem suited for it, AI allows humans to do amazing things. We can diagnose cancer at a glance or give a voice to those who cannot speak by simply applying the right algorithm in the correct way.
But AI isn’t a panacea or cure-all. In fact, when improperly applied, it’s a dangerous snake oil that should be avoided at all costs. To that end, I present six types of AI that I believe ethical developers should avoid.
First though, a brief explanation. I’m not passing judgment on developer intent or debating the core reasoning behind the development of these systems, but instead recognizing six areas where AI cannot provide a benefit to humans and is likely to harm us.
I’m not including military technology like autonomous weapons or AI-powered targeting systems because we do need debate on those technologies. And we’ve also intentionally left “knife technologies” off of this list. Those are techs such as DeepFakes which can arguably be used for good and evil, much like a knife can be used to chop vegetables or stab people.
Instead, I’ve focused on those technologies that distort the very problem they’re purported to solve. We’ll begin with the low hanging fruits: criminality and punishment.
Criminality
AI cannot determine the likelihood that a given individual, group of people, or specific population will commit a crime. Neither humans nor machines are psychic.
[Related: Predictive policing is a bigger scam than psychic detectives]
Predictive policing is racist. It uses historical data to predict where crime is most likely to occur based on past trends. If police visit a specific neighborhood more often than others and arrest people in that neighborhood regularly, an AI trained on data from that geographic area will determine that crime is more likely to happen in that neighborhood than others.
Put in another perspective: If you shop at Wal Mart exclusively for toilet paper and you’ve never purchased toilet paper from Amazon, you’re more likely to associate toilet paper with Wal Mart than Amazon. That doesn’t mean there’s more toilet paper at Wal Mart.
AI that attempts to predict criminality is fundamentally flawed because the vast majority of crimes go unnoticed. Developers are basically creating machines that validate whatever the cops have already done. They don’t predict crime, they just reinforce the false idea that over-policing low-income neighborhoods lowers crime. This makes the police look good.
But it doesn’t actually indicate which individuals in a society are likely to commit a crime. In fact, at best it just keeps an eye on those that’ve already been caught. At worst, these systems are a criminal’s best friend. The more they’re used, the more likely crime will perpetuate in areas where police presence is traditionally low.
Punishment
Algorithms cannot determine how likely a human is to commit a crime again after being convicted of a previous crime. See above, psychics do not exist. What a machine can do is take historical sentencing records and come to the mathematically sensible solution that people who are punished harshest tend to be the most recidivist and, thus, falsely indicate that Black people must be more likely to commit crimes than white people.
This is exactly what happens when developers use the wrong data for a problem. If you’re supposed to add 2 + 2, there’s no use for an apple in your equation. In this case, what that means is historical data on people who’ve committed crimes after release from the judicial system isn’t relevant to whether or not any specific individual will follow suit.
[Read: Why the criminal justice system should abandon algorithms]
People aren’t motivated to commit crimes because strangers they’ve never met were motivated to commit crimes upon release from custody. This information, how the general populace responds to release from incarceration, is useful for determining whether our justice system is actually rehabilitating people or not, but it cannot determine how likely a “Black male, 32, Boston, first offense” is to commit a post-conviction crime.
No amount of data can actually predict whether a human will commit a crime. It’s important to understand this because you can’t un-arrest, un-incarcerate, or un-traumatize a person who has been wrongfully arrested, imprisoned, or sentenced based on erroneous evidence generated from an algorithm.
Gender
Here’s a fun one. A company recently developed an algorithm that could allegedly determine someone’s gender from their name, email address, or social media handle. Sure, and I’ve got an algorithm that makes your poop smell like watermelon Jolly Ranchers (note: I do not. That’s sarcasm. Don’t email me.).
AI cannot determine a person’s gender from anything other than that person’s explicit description of their gender. Why? You’ll see a theme developing here: because psychics don’t exist.
Humans cannot look at other humans and determine their gender. We can guess, and we’re often correct, but let’s do a quick thought experiment:
If you lined up every human on the planet and looked at their faces to determine whether they were “male” or “female” how many would you get wrong? Do you think an AI is better at determining human gender in the margin cases where even you, a person who can read and everything, can’t get it right? Can you tell an intersex person by their face? Can you always tell what gender someone was assigned at birth by looking at their face? What if they’re Black or Asian?
Let’s simplify: even if your PhD is in gender studies and you’ve studied AI under Ian Goodfellow, you cannot build a machine that understands gender because humans themselves do not. You cannot tell every person’s gender, which means your machine will get some wrong. There are no domains where misgendering humans is beneficial, but there are myriad domains where doing so will cause direct harm to the humans who have been misgendered.
Any tool that attempts to predict human gender has no use other than as a weapon against the transgender, non-binary, and intersex communities.
Sexuality
Speaking of dangerous AI systems that have no possible positive use case: Gaydar is among the most offensive ideas in the machine learning world.
Artificial intelligence cannot predict a person’s sexuality because, you guessed it: psychics don’t exist. Humans cannot tell if other humans are gay or straight unless the subject of scrutiny expressly indicates exactly what their sexuality is.
[Read: The Stanford Gaydar is hogwash]
Despite the insistence of various members of the I’m-straight and I’m-gay crowds, human sexuality is far more complex than whether or not we’re born with gay face because our moms gave us different hormones, or if we’re adverse to heterosexual sexual encounters because… whatever it is that straight people think makes gay people gay these days.
In the year 2020 some scientists are still debating whether bisexual men exist. As an out pansexual, I can’t help but wonder if they’ll be debating my existence in another 20 or 30 years when they catch up to the fact that “gay and straight” as binary concepts have been outdated in the field of human psychology and sexuality since the 1950s. But I digress.
You cannot build a machine that predicts human sexuality because human sexuality is a social construct. Here’s how you can come to that same conclusion on your own:
Imagine a 30 year old person who has never had sex or been romantically attracted to anyone. Now imagine they fantasize about sex with women. A day later they have sex with a man. Now they fantasize about men. A day later they have sex with a woman. Now they fantasize about both. After a month, they haven’t had sex again and stop fantasizing. They never have sex again or feel romantically inclined towards another person. Are they gay, straight, or bisexual? Asexual? Pansexual?
That’s not up to you or any robot to decide. Does thinking about sex account for any part of your sexuality? Or are you “straight until you do some gay stuff?” How much gay stuff does someone have to do before they get to be gay? If you stop doing gay stuff can you ever be straight again?”
The very idea that a computer science expert is going to write an algorithm that can solve this for anyone is ludicrous. And it’s dangerous.
There is no conceivable good that can come from Gaydar AI. It’s only use is as a tool for discrimination.
Intelligence
AI cannot determine how intelligent a person is. I’m going to flip the script here because this has nothing to do with being psychic. When AI attempts to predict human intelligence it’s performing prestidigitation. It’s doing a magic trick and, like any good illusion, there’s no actual substance to it.
We can’t know a person’s intelligence unless we test it and, even then, there’s no universally recognized method of measuring pure human intelligence. Tests can be biased, experts dispute which questions are best, and nobody knows how to deal with hyperintelligent humans with mental disorders. Figuring out how smart a person is can’t be solved by a few algorithms.
So what do these AI systems do? They search for evidence of intelligence by comparing whatever data they’re given on a person to whatever model for intelligence the developers have come up with. For instance, they might determine that an intelligent person doesn’t use profanity as often as a non-intelligent person. In this instance, Dane Cook would be considered more intelligent than George Carlin.
That’s a comedic way of looking at it, but the truth is that there’s no positive use case for a robot that arbitrarily declares one human smarter than another. But there are plenty of ways these systems can be used to discriminate.
Potential
Ah yes, human potential. Here I want to focus on hiring algorithms, but this applies to any AI system designed to determine which humans, out of a pool, are more likely to succeed at a task, job, duty, or position than others.
Most major companies, in some form or another, use AI in their hiring process. These systems are almost always biased, discriminatory, and unethical. In the rare cases they aren’t, it’s where they seek out a specific, expressed, qualification.
If you design an AI to crawl thousands of job applications for “those who meet the minimum requirement of a college degree in computer science” with no other parameters… well, you could have done it quicker and cheaper with a non-AI system… but I guess that wouldn’t be discriminatory.
Otherwise, there’s no merit to developing AI hiring systems. Any data they’re trained on is either biased or useless. If you use data based on past successful applicants or industry-wide successful applicants, you’re entrenching the status quo and intentionally avoiding diversity.
The worst systems however, are the ones purported to measure a candidate’s “emotional intelligence” or “how good a fit” they’ll be. AI systems that parse applications and resumes for “positive” keywords and “negative” keywords as well as video systems that use “emotional recognition” to determine the best candidates are all inherently biased, and almost all of them are racist, sexist, ageist, and ableist.
AI cannot determine the best human candidate for a job, because people aren’t static concepts. You can’t send a human or a machine down to the store to buy a perfect HR fit. What these systems do is remind everyone that, traditionally, heterosexual, healthy, white men under the age of 55 is what most companies in the US and Europe hire, so it’s considered a safe bet to just keep doing that.
And there you have it, six incredibly popular areas of AI development – I’d estimate that there are hundreds of startups working on predictive policing and hiring algorithms alone – that should be placed on any ethical developer’s do not develop list.
Not because they could be used for evil, but because they cannot be used for good. Each of these six AI paradigms are united by subterfuge. They purport to solve an unsolvable problem with artificial intelligence and then deliver a solution that’s nothing more than alchemy.
Furthermore, in all six categories the binding factor is that they’re measured by an arbitrary percentage that some how indicates how “close” they are to “human level.” But “human level” in every single one of these six domains, means “our best guess.”
Our best guess is never good enough when the “problem” we’re solving is whether a specific human should be employed, free, or alive. It’s beyond the pale that anyone would develop an algorithm that served to only bypass human responsibility for a decision a robot is incapable of making ethically.
Published July 31, 2020 — 19:37 UTC