These days, it can be very hard to determine where to draw the boundaries around artificial intelligence. What it can and can’t do is often not very clear, as well as where it’s future is headed.
In fact, there’s also a lot of confusion surrounding what AI really is. Marketing departments have a tendency to somehow fit AI in their messaging and rebrand old products as “AI and machine learning.” The box office is filled with movies about sentient AI systems and killer robots that plan to conquer the universe. Meanwhile, social media is filled with examples of AI systems making stupid (and sometimes offending) mistakes.
“If it seems like AI is everywhere, it’s partly because ‘artificial intelligence’ means lots of things, depending on whether you’re reading science fiction or selling a new app or doing academic research,” writes Janelle Shane in You Look Like a Thing and I Love You, a book about how AI works.
Shane runs the famous blog AI Weirdness, which, as the name suggests, explores the “weirdness” of AI through practical and humorous examples. In her book, Shane taps into her years-long experience and takes us through many examples that eloquently show what AI—or more specifically deep learning—is and what it isn’t, and how we can make the most out of it without running into the pitfalls.
While the book is written for the layperson, it is definitely a worthy read for people who have a technical background and even machine learning engineers who don’t know how to explain the ins and outs of their craft to less technical people.
Dumb, lazy, greedy, and unhuman
In her book, Shane does a great job of explaining how deep learning algorithms work. From stacking up layers of artificial neurons, feeding examples, backpropagating errors, using gradient descent, and finally adjusting the network’s weights, Shane takes you through the training of deep neural networks with humorous examples such as rating sandwiches and coming up with “knock-knock who’s there?” jokes.
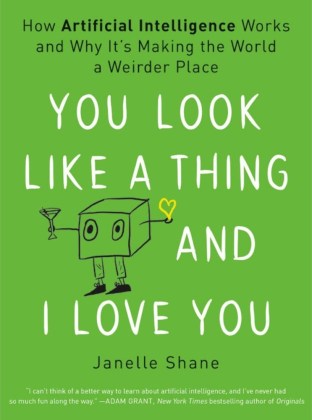
All of this helps understand the limits and dangers of current AI systems, which has nothing to do with super-smart terminator bots who want to kill all humans or software system planning sinister plots. “[Those] disaster scenarios assume a level of critical thinking and a humanlike understanding of the world that AIs won’t be capable of for the foreseeable future,” Shane writes.She uses the same context to explain some of the common problems that occur when training neural networks, such as class imbalance in the training data, algorithmic bias, overfitting, interpretability problems, and more.
Instead, the threat of current machine learning systems, which she rightly describes as narrow AI, is to consider it too smart and rely on it to solve a problem that is broader than its scope of intelligence. “The mental capacity of AI is still tiny compared to that of humans, and as tasks become broad, AIs begin to struggle,” she writes elsewhere in the book.
AI algorithms are also very unhuman and, as you will see in You Look Like a Thing and I Love You, they often find ways to solve problems that are very different from how humans would do it. They tend to ferret out the sinister correlations that humans have left in their wake when creating the training data. And if there’s a sneaky shortcut that will get them to their goals (such as pausing a game to avoid dying), they will use it unless explicitly instructed to do otherwise.
“The difference between successful AI problem solving and failure usually has a lot to do with the suitability of the task for an AI solution,” Shane writes in her book.
As she delves into AI weirdness, Shane sheds light on another reality about deep learning systems: “It can sometimes be a needlessly complicated substitute for a commonsense understanding of the problem.” She then takes us through a lot of other overlooked disciplines of artificial intelligence that can prove to be equally efficient at solving problems.
From stupid bots to human bots
In You Look Like a Thing and I Love You, Shane also takes care to explain some of the problems that have been created as a result of the widespread use of machine learning in different fields. Perhaps the best known is algorithmic bias, the intricate imbalances in AI’s decision-making which lead to discrimination against certain groups and demographics.
There are many examples where AI algorithms, using their own weird ways, discover and copy the racial and gender biases of humans and copy them in their decisions. And what makes it more dangerous is that they do it unknowingly and in an uninterpretable fashion.
“We shouldn’t see AI decisions as fair just because an AI can’t hold a grudge. Treating a decision as impartial just because it came from an AI is known sometimes as mathwashing or bias laundering,” Shane warns. “The bias is still there, because the AI copied it from its training data, but now it’s wrapped in a layer of hard-to-interpret AI behavior.”
This mindless replication of human biases becomes a self-reinforced feedback loop that can become very dangerous when unleashed in sensitive fields such as hiring decisions, criminal justice, and loan application.
“The key to all this may be human oversight,” Shane concludes. “Because AIs are so prone to unknowingly solving the wrong problem, breaking things, or taking unfortunate shortcuts, we need people to make sure their ‘brilliant solution’ isn’t a head-slapper. And those people will need to be familiar with the ways AIs tend to succeed or go wrong.”
Shane also explores several examples in which not acknowledging the limits of AI has resulted in humans being enlisted to solve problems that AI can’t. Also known as “The Wizard of Oz” effect, this invisible use of often-underpaid human bots is becoming a growing problem as companies try to apply deep learning to anything and everything and are looking for an excuse to put an “AI-powered” label on their products.
“The attraction of AI for many applications is its ability to scale to huge volumes, analyzing hundreds of images or transactions per second,” Shane writes. “But for very small volumes, it’s cheaper and easier to use humans than to build an AI.”
AI is not here to replace humans… yet
All the egg-shell-and-mud sandwiches, the cheesy jokes, the senseless cake recipes, the mislabeled giraffes, and all the other weird things AI does bring us to a very important conclusion. “AI can’t do much without humans,” Shane writes. “A far more likely vision for the future, even one with the widespread use of advanced AI technology, is one in which AI and humans collaborate to solve problems and speed up repetitive tasks.”
While we continue the quest toward human-level intelligence, we need to embrace current AI as what it is, not what we want it to be. “For the foreseeable future, the danger will not be that AI is too smart but that it’s not smart enough,” Shane writes. “There’s every reason to be optimistic about AI and every reason to be cautious. It all depends on how well we use it.”
This article was originally published by Ben Dickson on TechTalks, a publication that examines trends in technology, how they affect the way we live and do business, and the problems they solve. But we also discuss the evil side of technology, the darker implications of new tech and what we need to look out for. You can read the original article here.
Published July 18, 2020 — 13:00 UTC