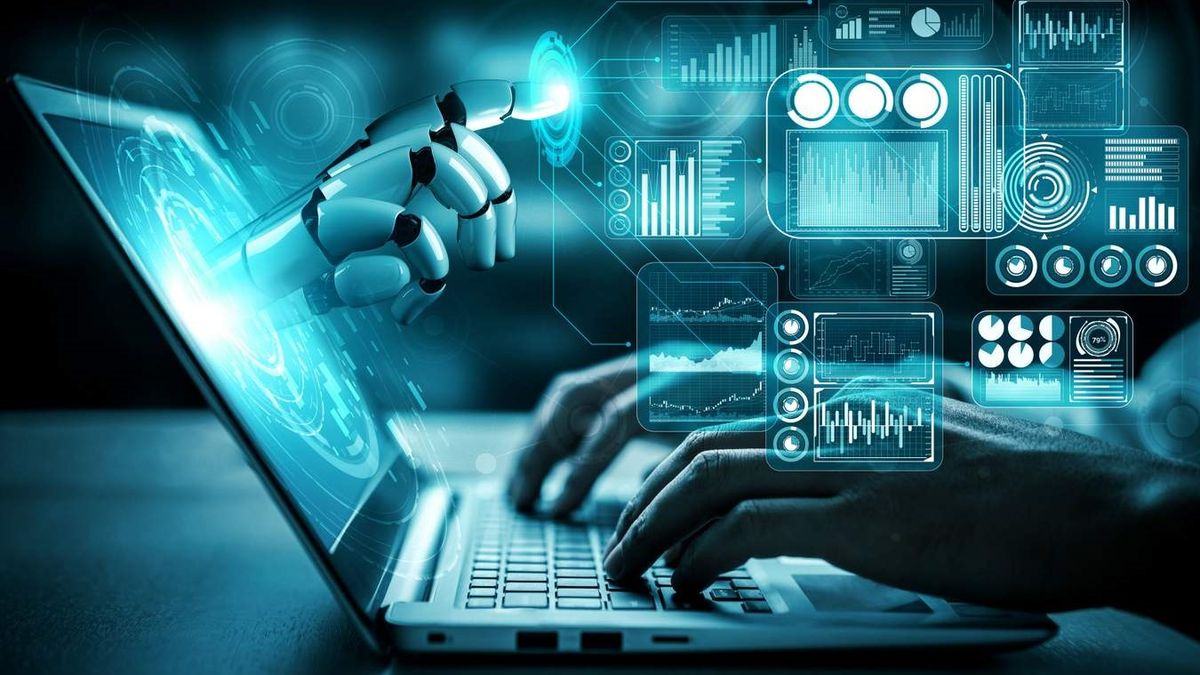
Technology has evolved significantly over the past few years, thanks in large part to breakthroughs in AI. Around 51 percent of businesses now use it for cybersecurity and fraud management because it can catch a potential breach before it causes trouble.
However, the effectiveness of AI can make people too reliant on this technology. The complete trust in artificial intelligence led to 77 percent of these companies discovering a breach in their AI system. As technology continuously changes, these issues are bound to happen again, especially if left unsupervised.
With that said, this doesn’t mean companies should avoid using AI for their cybersecurity needs. There is no doubt that AI can be an asset when it is used correctly. Instead, companies should be using it to augment human intelligence rather than replace it. Without including human input and oversight to the security formula, the chances of creating a blind spot can be very high.
Chief Marketing Officer at Kualitatem.
The issue of AI bias in cybersecurity
AI systems can easily detect a threat within seconds, because it can process present data extremely fast. The main issue is that training AI takes a lot of time – sometimes, it can take months for the AI system to fully grasp a new procedure. For instance, ChatGPT draws most of its data from information before 2023. The same applies to most AI tools because they need constant training and updating to keep their information accurate.
With the increase in cybersecurity threats, this can be quite problematic. As hackers create new and different ways to break through security systems, AI technology that is not up to date might not know how to react or miss a threat entirely.
In this scenario, human involvement is necessary because people can use their intuition and experience to determine whether or not there is a real cyber security threat. Without this human-centric skill, relying entirely on AI can cause false positives and negatives, which can lead to damaging cyber security breaches or wasting company resources.
Cultural bias can impact training data
Some AI systems can be trained with new data every week, ensuring they remain current most of the time. However, as diligent as the trainers may be, there is still the risk of cultural biases in the trained data. For instance, the U.S. has been at the forefront of some of the latest advances in cybersecurity. The problem is that it’s been done entirely in English so AI systems might miss threats coming from non-English speaking regions.
To avoid this problem, it’s critical to test systems and programs that use a culturally diverse set of AI tools and human involvement to cover potential blind spots.
Challenges of algorithmic bias
AI systems rely on the data they’ve been trained with to take a particular course of action. If that data is incomplete or there are flaws in the algorithm, the chances are high that it can lead to false positives and negatives, along with inaccuracies.
When this happens, the AI can start hallucinating, presenting courses of action and prompts that appear logical but are ethically incorrect. If the system was set to follow the AI’s direction without human involvement, the consequences could be major and time-consuming for the company.
These hallucinations can happen at any time, but they can also be avoided. For instance, humans can curate and validate the information regularly, ensuring everything is complete and up to date. The human side can also provide intuition, finding potential biases that could otherwise compromise the security algorithm.
AI is generally as good as the information that it has been fed, which is why it requires constant supervision. Should it be left alone for extended periods, the algorithm can become outdated and make decisions that are not current. The human brain should bring the innovative aspect that AI often seems to lack.
The matter of cognitive bias in AI tuning
AI systems take complex details from a large data pool to make an informed, non-emotional decision. However, the issue is that the data provided to the AI also comes from humans. Aside from their knowledge, the AI can also absorb their potential biases or mimic their lack of knowledge. In the end, AI systems are like a sponge: if the data trainer has biases, there is a good chance that the artificial mind will also have them.
For example, let’s say you are creating a security program preventing cyber-criminals from accessing your database. However, you have no knowledge in the cyber-security area; you just have the computer skills to put together a good algorithm. This lack of knowledge could be reflected in the written algorithm when predicting how a cyber-criminal might attack.
A diversified team is generally recommended to prevent that from happening. Not only can they complete the data pool, but they can also catch certain evasion techniques that might otherwise bypass the AI system. This could significantly reduce potential breaches and protect one’s system from a hidden threat.
The bottom line
In the end, while AI systems can be a significant asset in reducing the workload in cybersecurity, they cannot work independently. To ensure companies are fully protected from cyber security threats, AI should be used to augment human intelligence rather than replace it. This way, costly and time-consuming mistakes can be prevented in the long run.
We’ve featured the best encryption software.
This article was produced as part of TechRadarPro’s Expert Insights channel where we feature the best and brightest minds in the technology industry today. The views expressed here are those of the author and are not necessarily those of TechRadarPro or Future plc. If you are interested in contributing find out more here: https://www.techradar.com/news/submit-your-story-to-techradar-pro
Services Marketplace – Listings, Bookings & Reviews