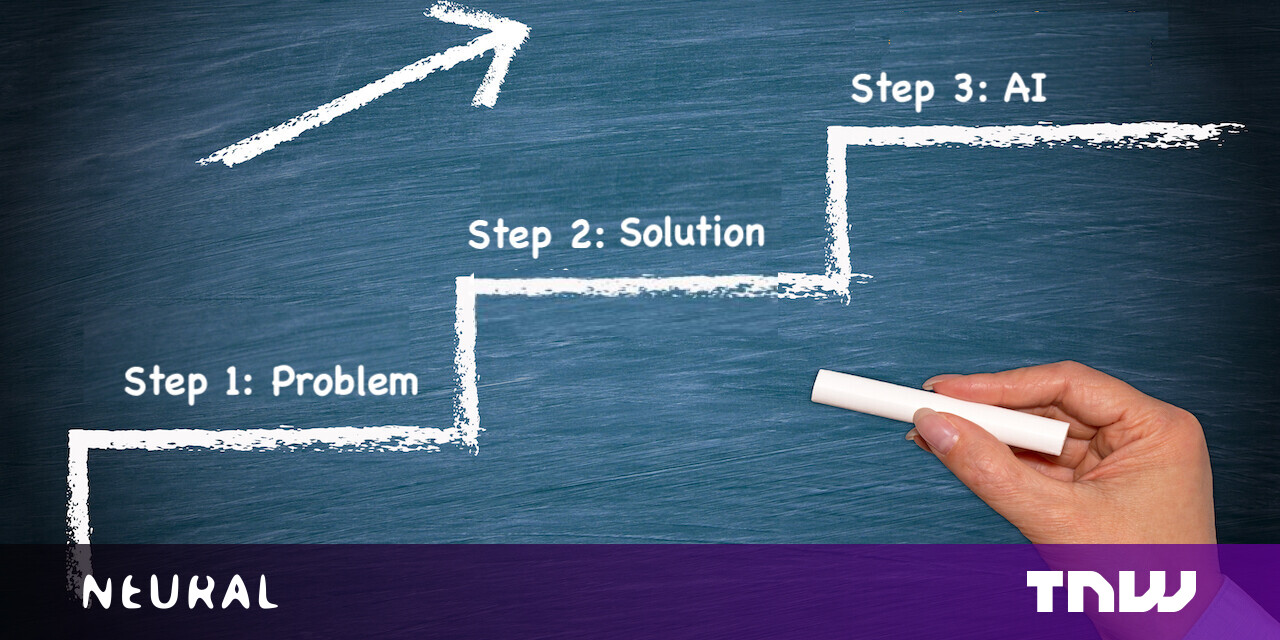
Artificial intelligence has become a buzzword in the tech industry. Companies are eager to present themselves as “AI-first” and use the terms “AI,” “machine learning,” and “deep learning” abundantly in their web and marketing copy.
What are the effects of the current hype surrounding AI? Is it just misleading consumers and end-users or is it also affecting investors and regulators? How is it shaping the mindset for creating products and services? How is the merging of scientific research and commercial product development feeding into the hype?
These are some of the questions that Richard Heimann, Chief AI Officer at Cybraics, answers in his new book Doing AI. Heimann’s main message is that when AI itself becomes our goal, we lose sight of all the important problems we must solve. And by extension, we draw the wrong conclusions and make the wrong decisions.
Machine learning, deep learning, and all other technologies that fit under the umbrella term “AI” should be considered only after you have well-defined goals and problems, Heimann argues. And this is why being AI-first means doing AI last.
One of the themes that Heimann returns to in the book is having the wrong focus. When companies talk about being “AI-first,” their goal becomes to somehow integrate the latest and greatest advances in AI research into their products (or at least pretend to do so). When this happens, the company starts with the solution and then tries to find a problem to solve with it.
Perhaps a stark example is the trend surrounding large language models, which are making a lot of noise in mainstream media and are being presented as general problem-solvers in natural language processing. While these models are truly impressive, they are not a silver bullet. In fact, in many cases, when you have a well-defined problem, a simpler model or even a regular expression or rule-based program can be more reliable than GPT-3.
“We interpret AI-first as though we ought to literally become solution-first without knowing why. What’s more is that we conceptualize an abstract, idealized solution that we place before problems and customers without fully considering whether it is wise to do so, whether the hype is true, or how solution-centricity impacts our business,” Heimann writes in Doing AI.
This is a pain point that I’ve encountered time and again in how companies try to pitch their products. I often read through a bunch of (sometimes self-contradicting) AI jargon, trying hard to find out what kind of a problem the company solves. Sometimes, I find nothing impressive.
“Anyone talking about AI without the support of a problem is probably not interested in creating a real business or has no idea what a business signifies,” Heimann told TechTalks. “Perhaps these wannapreneurs are looking for a strategic acquisition. If your dream is to be acquired by Google, you don’t always need a business. Google is one and doesn’t need yours. However, the fact that Google is a business should not be overlooked.”
The AI hype has attracted interest and funding to the field, providing startups and research labs with plenty of money to chase their dreams. But it has also had adverse effects. For one thing, using the ambiguous, anthropomorphic, and vaguely defined term “AI” sets high expectations in clients and users and causes confusion. It can also drive companies into overlooking more affordable solutions and waste resources on unnecessary technology.
“What is important to remember is that AI is not some monolith. It means different things to different people,” Heimann said. “It cannot be said without confusing everyone. If you’re a manager and say ‘AI,’ you have created external goals for problem-solvers. If you say ‘AI’ without a connection to a problem, you will create misalignments because staff will find problems suitable for some arbitrary solution.”
Academic AI research is focused on pushing the boundaries of science. Scientists study cognition, brain, and behavior in animals and humans to find hints about creating artificial intelligence. They use ImageNet, COCO, GLUE, Winograd, ARC, board games, video games, and other benchmarks to measure progress on AI. Although they know that their findings can serve humankind in the future, they are not worried about whether their technology will be commercialized or productized in the next few months or years.
Applied AI, on the other hand, aims to solve specific problems and ship products to the market. Developers of applied AI systems must meet memory and computational constraints imposed by the environment. They must conform to regulations and meet safety and robustness standards. They measure success in terms of audience, profits, and losses, customer satisfaction, growth, scalability, etc. In fact, in product development, machine learning and deep learning (and any other AI technology) become one of the many tools you use to solve customer problems.
In recent years, especially as commercial entities and big tech companies have taken the lead in AI research, the lines between research and applications have blurred. Today, companies like Google, Facebook, Microsoft, and Amazon account for much of the money that goes into AI research. Consequently, their commercial goals affect the directions that AI research takes.
“The aspiration to solve everything, instead of something, is the summit for insiders, and it’s why they seek cognitively plausible solutions,” Heimann writes in Doing AI. “But that does not change the fact that solutions cannot be all things to all problems, and, whether we like it or not, neither can business. Virtually no business requires solutions that are universal, because business is not universal in nature and often cannot achieve goals ‘in a wide range of environments.’”
An example is DeepMind, the UK-based AI research lab that was acquired by Google in 2014. DeepMind’s mission is to create safe artificial general intelligence. At the same time, it has a duty to turn in profits for its owner.
The same can be said of OpenAI, another research lab that chases the dream of AGI. But being mostly funded by Microsoft, OpenAI must find a balance between scientific research and developing technologies that can be integrated into Microsoft’s products.
“The boundaries [between academia and business] are increasingly difficult to recognize and are complicated by economic factors and motivations, disingenuous behavior, and conflicting goals,” Heimann said. “This is where you see companies doing research and publishing papers and behaving similarly to traditional academic institutions to attract academically-minded professionals. You also find academics who maintain their positions while holding industry roles. Academics make inflated claims and create AI-only businesses that solve no problem to grab cash during AI summers. Companies make big claims with academic support. This supports human resource pipelines, generally company prestige, and impacts the ‘multiplier effect.’”
Time and again, scientists have discovered that solutions to many problems don’t necessarily require human-level intelligence. Researchers have managed to create AI systems that can master chess, go, programming contests, and science exams without reproducing the human reasoning process.
These findings often create debates around whether AI should simulate the human brain or aim at producing acceptable results.
“The question is relevant because AI doesn’t solve problems in the same way as humans,” Heimann said. “Without human cognition, these solutions will not solve any other problem. What we call ‘AI’ is narrow and only solves problems they were intended to solve. That means business leaders still need to find problems that matter and either find the right solution or design the right solution to solve those problems.”
Heimann also warned that AI solutions that do not act like humans will fail in unique ways that are unlike humans. This has important implications for safety, security, fairness, trustworthiness, and many other social issues.
“It necessarily means we should use ‘AI’ with discretion and never on simple problems that humans could solve easily or when the cost of error is high, and accountability is required,” Heimann said. “Again, this brings us back to the nature of the problem we want to solve.”
In another sense, the question of whether AI should simulate the human brain lacks relevance because most AI research cares very little about cognitive plausibility or biological plausibility, Heimann believes.
“I often hear business-minded people espouse nonsense about artificial neural networks being ‘inspired by,…’ or ‘roughly mimic’ the brain,” he said. “The neuronal aspect of artificial neural networks is just a window dressing for computational functionalism that ignores all differences between silicon and biology anyway. Aside from a few counterexamples, artificial neural network research still focuses on functionalism and does not care about improving neuronal plausibility. If insiders generally don’t care about bridging the gap between biological and artificial neural networks, neither should you.”
In Doing AI, Heimann stresses that to solve sufficiently complex problems, we may use advanced technology like machine learning, but what that technology is called means less than why we used it. A business’s survival doesn’t rely on the name of a solution, the philosophy of AI, or the definition of intelligence.
He writes: “Rather than asking if AI is about simulating the brain, it would be better to ask, ‘Are businesses required to use artificial neural networks?’ If that is the question, then the answer is no. The presumption that you need to use some arbitrary solution before you identify a problem is solution guessing. Although artificial neural networks are very popular and almost perfect in the narrow sense that they can fit complex functions to data—and thus compress data into useful representations—they should never be the goal of business, because approximating a function to data is rarely enough to solve a problem and, absent of solving a problem, never the goal of business.”
When it comes to developing products and business plans, the problem comes first, and the technology follows. Sometimes, in the context of the problem, highlighting the technology makes sense. For example, a “mobile-first” application suggests that it addresses a problem that users mainly face when they’re not sitting behind a computer. A “cloud-first” solution suggests that storage and processing are mainly done in the cloud to make the same information available across multiple devices or to avoid overloading the computational resources of end-user devices. (It is worth noting that those two terms also became meaningless buzzwords after being overused. They were meaningful in the years when companies were transitioning from on-premise installations to the cloud and from web to mobile. Today, every application is expected to be available on mobile and to have a strong cloud infrastructure.)
But what does “AI-first” say about the problem and context of the application and the problem it solves?
“AI-first is an oxymoron and an ego trip. You cannot do something before you understand the circumstances that make it necessary,” Heimann said. “AI strategies, such as AI-first, could mean anything. Business strategy is too broad when it includes everything or things it shouldn’t, like intelligence. Business strategy is too narrow when it fails to include things that it should, like mentioning an actual problem or a real-world customer. Circular strategies are those in which a solution defines a goal, and the goal defines that solution.
“When you lack problem-, customer-, and market-specific information, teams will fill in the blanks and work on whatever they think of when they think of AI. Nevertheless, you are unlikely to find a customer inside an abstract solution like ‘AI.’ Therefore, artificial intelligence cannot be a business goal, and when it is, strategy is more complex verging on impossible.”
This article was originally written by Ben Dickson and published by Ben Dickson on TechTalks, a publication that examines trends in technology, how they affect the way we live and do business, and the problems they solve. But we also discuss the evil side of technology, the darker implications of new tech, and what we need to look out for. You can read the original article here.