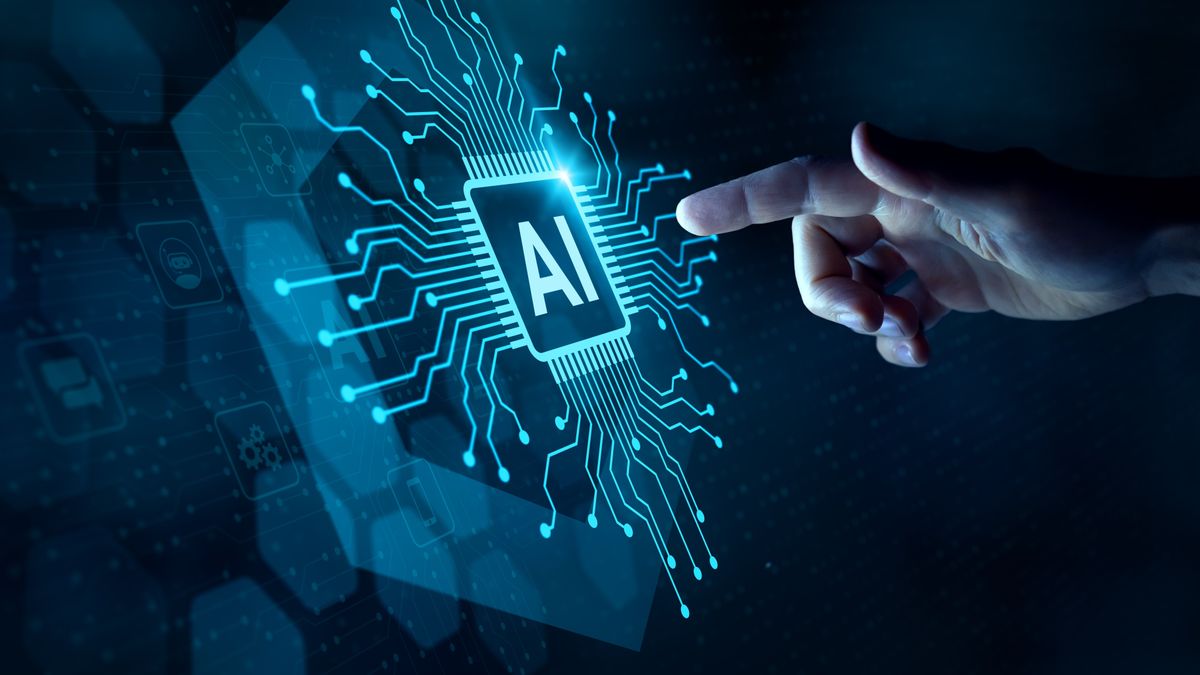
Today, it’s common to see tech and data teams coming under significant pressure from their organization’s C-suite to ‘roll-out’ AI, and fast. AI, and specifically generative AI, is the technology of the moment and many C-suite leaders want to see progress being made in this area immediately; Gartner found that 62% of CFOs and 58% of CEOs believe that AI will have the most significant impact on their industries in the next three years.
However, tech teams are all too aware that speed does not always equal success. In fact, too fast can actually hamper eventual progress.. So, how can technical teams roll out AI in a safe way, whilst also meeting the expectations of the C-suite?
Lead Data Strategist at Databricks.
The risks of a rushed rollout
Firstly, it’s important to understand what risks are associated with implementing AI without a proper roadmap, and too fast. One is over-implementation, which can lead to duplicated efforts as two or more tools are used essentially to do the same thing – in turn leading to wasted resources and unnecessary costs. That’s not to say that harnessing the energy of that eagerness is a bad thing, it’s just that too much misdirected energy can lead to wasted effort.
One of the things generative AI has really highlighted is the importance of data and information quality, and what the consequences might be of bad data. If a model hallucinates and gives incorrect answers which are then acted upon, there could be huge repercussions. For example, an employee could look to find out which deals they are able to offer to customers. If the model generates an incorrect answer, and the employee follows through with this offer, the business’ bottom line takes a hit. Additionally, if incorrect information is provided and supplied to stakeholders, reputation and trust could be damaged. More than half of AI users already admit to finding it hard to get what they want out of AI, and when applied to a business context – the risks multiply.
Not only is the data key, but the models being used too. Particularly for organizations who are heavily regulated, but it is a massive consideration for all. If decisions are being made based on the output of a certain model, it’s vital that the outcome can be replicated and traced. So, a key challenge is ensuring that a model is reliable, consistent, and safe. Here, the data being used to train a model is hugely significant – data is the fuel of AI, and so its quality determines how accurate and trustworthy a model can be. Implementing too fast can mean that key steps are missed, such as ensuring high quality, accurate data is in use. Getting this step wrong could mean that organizations find themselves dealing with the fallout further down the line. Technical teams know this, but communicating this to the C-suite can often be a real challenge.
Controlled AI experimentation – Finding the middle ground
So, what if there is a middle ground that both sides of the aisle can meet on? There is, and it lies in AI experimentation. Recent MIT research found that 56% more companies are logging experimental models compared to a year ago, and rightly so. Experimentation has huge potential benefits and can be used to work on business ‘pain points’, bringing business and tech closer together. For one, experimenting with generative AI can help identify the most valuable use cases that are going to have the biggest impact on organizations.
Experimentation also involves putting AI into production in a safe, controlled environment, where issues can be spotted and resolved. For instance, if a model is giving employees inaccurate answers, the developers can turn to the data the model is being trained upon to address these issues before it is rolled out officially. Experimentation can also help organizations identify what governance needs to be in place; does there need to an operating model or, at the very least, a coordinated set of of hand-offs between teams to govern the end-to-end generative AI cycle? Experimentation can also highlight where there is an abundance of skills, as well as where they gaps are, in turn enabling organizations to plan for any future upskilling programs. Then finally, and possibly most significantly, experimentation can spotlight where there might be data issues which need addressing to totally productionize any generative AI model.
Experimenting in this way ticks the box for both the C-suite, and technical teams. From the C-suite’s perspective, they are seeing action when it comes to AI, confirming that the company is not falling behind. For technical teams, they have more control over the pace and quality of the roll-out. However, for experimentation to be truly effective, there’s a few things technical teams need to make sure of.
Leveraging technology for safe AI experimentation
Accessing or unifying data in one place is a key enabler for generative AI. Platforms such as a Data Intelligence Platform, can unify data and models, providing organizations with one place to go to access the data they need for their generative AI use-cases. Finding the right AI tools that enable a safe place to experiment will also be key, allowing end-users to access and validate various LLMs to select the most appropriate one for their use cases. Finally, having proper governance in place will allow organizations to monitor and manage access to data and models, as well as performance and lineage – all of which are integral to the success of generative AI.
In the high-stakes race to harness the power of AI, finding a balance between speed and caution is paramount. The pressure from the C-suite to deploy AI rapidly is understandable, however, as technical teams know all too well, a hasty implementation can lead to significant pitfalls. AI experimentation offers a pragmatic middle ground, allowing companies to meet the urgent demands of leadership while ensuring robust, reliable AI systems which can truly drive transformative success.
We’ve featured the best AI phone.
This article was produced as part of TechRadarPro’s Expert Insights channel where we feature the best and brightest minds in the technology industry today. The views expressed here are those of the author and are not necessarily those of TechRadarPro or Future plc. If you are interested in contributing find out more here: https://www.techradar.com/news/submit-your-story-to-techradar-pro
Services Marketplace – Listings, Bookings & Reviews